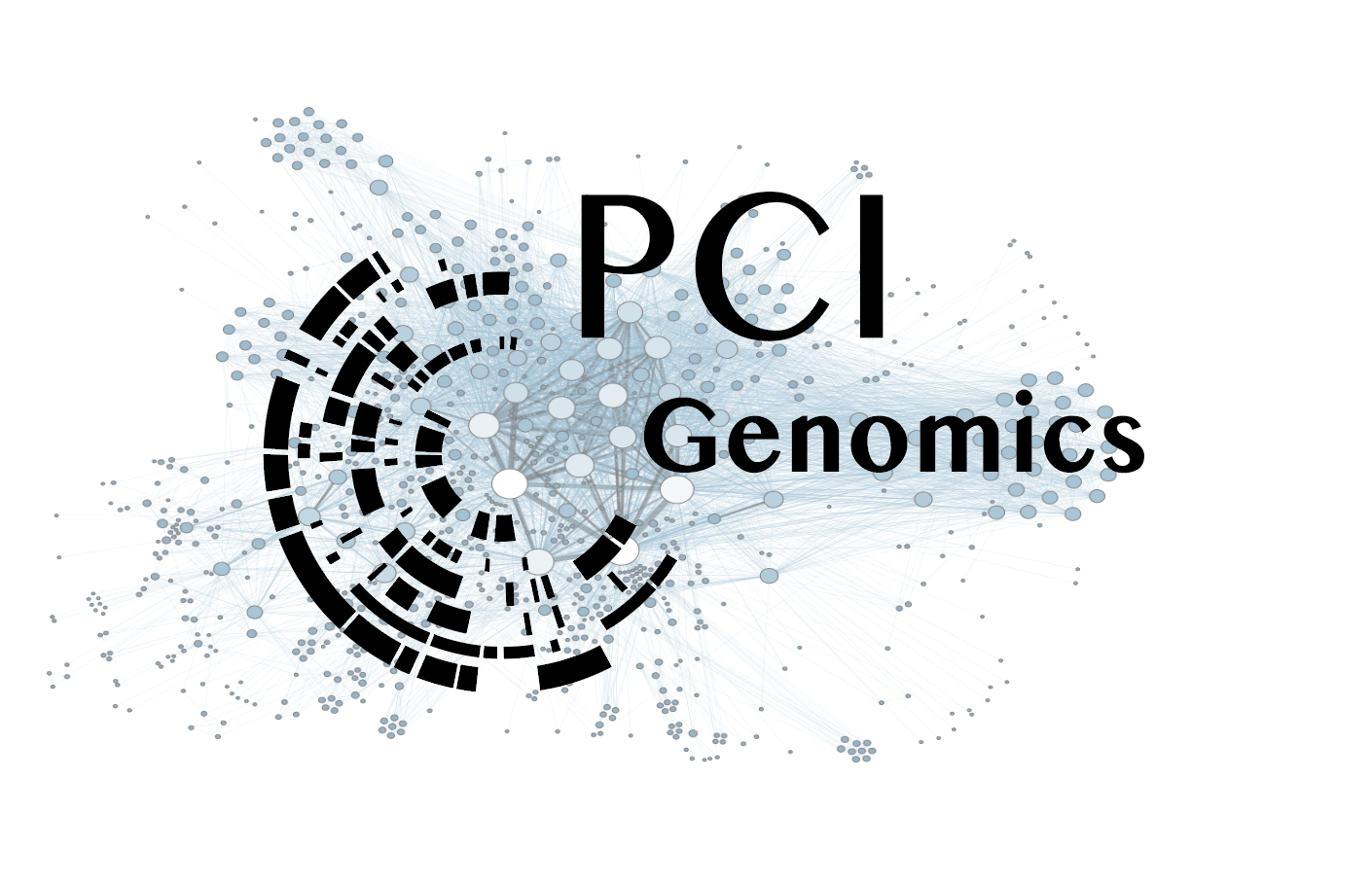
A hitchhiker’s guide to DNA-based microbiome analysis
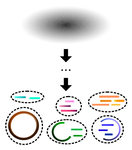
A primer and discussion on DNA-based microbiome data and related bioinformatics analyses
Abstract
Recommendation: posted 01 May 2021, validated 05 May 2021
Ionescu, D. (2021) A hitchhiker’s guide to DNA-based microbiome analysis. Peer Community in Genomics, 100049. https://doi.org/10.24072/pci.genomics.100049
Recommendation
In the last two decades, microbial research in its different fields has been increasingly focusing on microbiome studies. These are defined as studies of complete assemblages of microorganisms in given environments and have been benefiting from increases in sequencing length, quality, and yield, coupled with ever-dropping prices per sequenced nucleotide. Alongside localized microbiome studies, several global collaborative efforts have emerged, including the Human Microbiome Project [1], the Earth Microbiome Project [2], the Extreme Microbiome Project, and MetaSUB [3].
Coupled with the development of sequencing technologies and the ever-increasing amount of data output, multiple standalone or online bioinformatic tools have been designed to analyze these data. Often these tools have been focusing on either of two main tasks: 1) Community analysis, providing information on the organisms present in the microbiome, or 2) Functionality, in the case of shotgun metagenomic data, providing information on the metabolic potential of the microbiome. Bridging between the two types of data, often extracted from the same dataset, is typically a daunting task that has been addressed by a handful of tools only.
The extent of tools and approaches to analyze microbiome data is great and may be overwhelming to researchers new to microbiome or bioinformatic studies. In their paper “A primer and discussion on DNA-based microbiome data and related bioinformatics analyses”, Douglas and Langille [4] guide us through the different sequencing approaches useful for microbiome studies. alongside their advantages and caveats and a selection of tools to analyze these data, coupled with examples from their own field of research.
Standing out in their primer-style review is the emphasis on the coupling between taxonomic/phylogenetic identification of the organisms and their functionality. This type of analysis, though highly important to understand the role of different microorganisms in an environment as well as to identify potential functional redundancy, is often not conducted. For this, the authors identify two approaches. The first, using shotgun metagenomics, has higher chances of attributing a function to the correct taxon. The second, using amplicon sequencing of marker genes, allows for a deeper coverage of the microbiome at a lower cost, and extrapolates the amplicon data to close relatives with a sequenced genome. As clearly stated, this approach makes the leap between taxonomy and functionality and has been shown to be erroneous in cases where the core genome of the bacterial genus or family does not encompass the functional diversity of the different included species. This practice was already common before the genomic era, but its accuracy is improving thanks to the increasing availability of sequenced reference genomes from cultures, environmentally picked single cells or metagenome-assembled genome.
In addition to their description of standalone tools useful for linking taxonomy and functionality, one should mention the existence of online tools that may appeal to researchers who do not have access to adequate bioinformatics infrastructure. Among these are the Integrated Microbial Genomes and Microbiomes (IMG) from the Joint Genome Institute [5], KBase [6] and MG-RAST [7].
A second important point arising from this review is the need for standardization in microbiome data analyses and the complexity of achieving this. As Douglas and Langille [4] state, this has been previously addressed, highlighting the variability in results obtained with different tools. It is often the case that papers describing new bioinformatic tools display their superiority relative to existing alternatives, potentially misleading newcomers to the field that the newest tool is the best and only one to be used. This is often not the case, and while benchmarking against well-defined datasets serves as a powerful testing tool, “real-life” samples are often not comparable. Thus, as done here, future primer-like reviews should highlight possible cross-field caveats, encouraging researchers to employ and test several approaches and validate their results whenever possible.
In summary, Douglas and Langille [4] offer both the novice and experienced researcher a detailed guide along the paths of microbiome data analysis, accompanied by informative background information, suggested tools with which analyses can be started, and an insightful view on where the field should be heading.
References
[1] Turnbaugh PJ, Ley RE, Hamady M, Fraser-Liggett CM, Knight R, Gordon JI (2007) The Human Microbiome Project. Nature, 449, 804–810. https://doi.org/10.1038/nature06244
[2] Gilbert JA, Jansson JK, Knight R (2014) The Earth Microbiome project: successes and aspirations. BMC Biology, 12, 69. https://doi.org/10.1186/s12915-014-0069-1
[3] Mason C, Afshinnekoo E, Ahsannudin S, Ghedin E, Read T, Fraser C, Dudley J, Hernandez M, Bowler C, Stolovitzky G, Chernonetz A, Gray A, Darling A, Burke C, Łabaj PP, Graf A, Noushmehr H, Moraes s., Dias-Neto E, Ugalde J, Guo Y, Zhou Y, Xie Z, Zheng D, Zhou H, Shi L, Zhu S, Tang A, Ivanković T, Siam R, Rascovan N, Richard H, Lafontaine I, Baron C, Nedunuri N, Prithiviraj B, Hyat S, Mehr S, Banihashemi K, Segata N, Suzuki H, Alpuche Aranda CM, Martinez J, Christopher Dada A, Osuolale O, Oguntoyinbo F, Dybwad M, Oliveira M, Fernandes A, Oliveira M, Fernandes A, Chatziefthimiou AD, Chaker S, Alexeev D, Chuvelev D, Kurilshikov A, Schuster S, Siwo GH, Jang S, Seo SC, Hwang SH, Ossowski S, Bezdan D, Udekwu K, Udekwu K, Lungjdahl PO, Nikolayeva O, Sezerman U, Kelly F, Metrustry S, Elhaik E, Gonnet G, Schriml L, Mongodin E, Huttenhower C, Gilbert J, Hernandez M, Vayndorf E, Blaser M, Schadt E, Eisen J, Beitel C, Hirschberg D, Schriml L, Mongodin E, The MetaSUB International Consortium (2016) The Metagenomics and Metadesign of the Subways and Urban Biomes (MetaSUB) International Consortium inaugural meeting report. Microbiome, 4, 24. https://doi.org/10.1186/s40168-016-0168-z
[4] Douglas GM, Langille MGI (2021) A primer and discussion on DNA-based microbiome data and related bioinformatics analyses. OSF Preprints, ver. 4 peer-reviewed and recommended by Peer Community In Genomics. https://doi.org/10.31219/osf.io/3dybg
[5] Chen I-MA, Markowitz VM, Chu K, Palaniappan K, Szeto E, Pillay M, Ratner A, Huang J, Andersen E, Huntemann M, Varghese N, Hadjithomas M, Tennessen K, Nielsen T, Ivanova NN, Kyrpides NC (2017) IMG/M: integrated genome and metagenome comparative data analysis system. Nucleic Acids Research, 45, D507–D516. https://doi.org/10.1093/nar/gkw929
[6] Arkin AP, Cottingham RW, Henry CS, Harris NL, Stevens RL, Maslov S, Dehal P, Ware D, Perez F, Canon S, Sneddon MW, Henderson ML, Riehl WJ, Murphy-Olson D, Chan SY, Kamimura RT, Kumari S, Drake MM, Brettin TS, Glass EM, Chivian D, Gunter D, Weston DJ, Allen BH, Baumohl J, Best AA, Bowen B, Brenner SE, Bun CC, Chandonia J-M, Chia J-M, Colasanti R, Conrad N, Davis JJ, Davison BH, DeJongh M, Devoid S, Dietrich E, Dubchak I, Edirisinghe JN, Fang G, Faria JP, Frybarger PM, Gerlach W, Gerstein M, Greiner A, Gurtowski J, Haun HL, He F, Jain R, Joachimiak MP, Keegan KP, Kondo S, Kumar V, Land ML, Meyer F, Mills M, Novichkov PS, Oh T, Olsen GJ, Olson R, Parrello B, Pasternak S, Pearson E, Poon SS, Price GA, Ramakrishnan S, Ranjan P, Ronald PC, Schatz MC, Seaver SMD, Shukla M, Sutormin RA, Syed MH, Thomason J, Tintle NL, Wang D, Xia F, Yoo H, Yoo S, Yu D (2018) KBase: The United States Department of Energy Systems Biology Knowledgebase. Nature Biotechnology, 36, 566–569. https://doi.org/10.1038/nbt.4163
[7] Wilke A, Bischof J, Gerlach W, Glass E, Harrison T, Keegan KP, Paczian T, Trimble WL, Bagchi S, Grama A, Chaterji S, Meyer F (2016) The MG-RAST metagenomics database and portal in 2015. Nucleic Acids Research, 44, D590–D594. https://doi.org/10.1093/nar/gkv1322
The recommender in charge of the evaluation of the article and the reviewers declared that they have no conflict of interest (as defined in the code of conduct of PCI) with the authors or with the content of the article. The authors declared that they comply with the PCI rule of having no financial conflicts of interest in relation to the content of the article.
Evaluation round #2
DOI or URL of the preprint: 10.31219/osf.io/3dybg
Version of the preprint: 2
Author's Reply, 16 Apr 2021
Decision by Danny Ionescu
, posted 12 Apr 2021
Dear Drs. Douglas and Langille,
Thank you for revising your manuscript according to the reviewer's and my suggestions.
I would like to ask for several minor changes prior to recommending your paper.
1) On line 73 you write "First..." but there is never "Second". Probably this should come on line 83. Please add "Second" or rephrase "First".
2) In line 1163 you have "hereafter 16S". I think this can be replaced by the "hereafter 16S sequencing" in line 305. As also there it seems you mean to replace the 16S rRNA gene with the shorter 16S.
The following requests were made by the PCI management board with regards to the original version and I could not see these amendments in the revised version:
1) Authors must have no financial conflict of interest relating to the article. The article must contain a "Conflict of interest disclosure" paragraph before the reference section containing this sentence: "The authors of this article declare that they have no financial conflict of interest with the content of this article.";
2) This disclosure has to be completed by a sentence indicating that some of the authors are PCI recommenders: “XY is one of the PCI Genomics recommenders.”
I believe that other requests made by the board regarding data or code availability are not relevant for a review-type manuscript.
Following these minor changes/additions, I am looking forward to recommending your manuscript.
Best wishes,
Danny Ionescu
Evaluation round #1
DOI or URL of the preprint: 10.31219/osf.io/3dybg
Author's Reply, 06 Apr 2021
Decision by Danny Ionescu
, posted 22 Mar 2021
Dear Dr. Douglas and Langille,
Thank you for submitting your manuscript to be reviewed by PCI members.
I have obtained 3 independent reviews for your manuscript and have further reviewed the manuscript myself. The attached file contains all comments and suggestions.
Generally, the reviewers and I found the manuscript relevant and interesting. I do agree with the first reviewer that occasionally there are distracting facts, of added value, that reduce the usability of the manuscript as a "guide for the novice". I do not suggest removing these but rather relocating them to a box. For example - the necessary traits of a marker gene are good to know, but realistically, most people embarking on the metabarcoding adventure will initially embrace known markers.
With this respect, I feel that the paper can be made somewhat more concise.
As a primer - I suggest adding a glossary and to minimize abbreviations as much as possible.
Last, it is evident that the authors come from the field of human microbiome and so are most of the examples. I suggest adding a paragraph where this is specified clearly, explaining how the provided guidelines can be applied to microbial ecology in other types of environments (e.g. water, soil, biofilms, etc).
I hope the provided suggestions are useful,
looking forward to reading your revised version,
Best wishes,
Danny Ionescu
Download recommender's annotations
Reviewed by anonymous reviewer 1, 16 Mar 2021
In this article, the authors propose an overview of the use of different approaches for microbiome data analyses, the questions that can be tackled using them, and their respective limitations. A particular focus is provided on the bioinformatics aspects, including an overview of the diversity of the most popular tools, and under which conditions/for which specific purposes they could be better used. Taxonomic and functional assignations tools are thoroughly discussed. And the crucial question of how to integrate the taxonomic and functional aspects. How marker-gene and shotgun metagenomic sequences (MGS) data are currently linked is exposed and the limitations of different approaches given. How the two approaches can lead to contradicting results, as well as the recurrent problem of reproducibility on microbiome data when using different bioinformatics pipelines are thoroughly discussed. Some interesting leads on how to make the field of microbiome biology more robust are given.
The paper is very well-written and thorough on several aspects, including by explaining the main trends in “DNA-based microbiome data” analyses. It is very interesting both from the level of technical details that are given, and from the fact that it does the synthesis of the current major pitfalls in microbiome studies. I particularly enjoyed the “Overview” and the last section on “Current state of the integration of taxonomic and functional data types”. As such, beyond proposing a view of the current state-of-the-art, I think this primer paper should contribute to the reflexion on what good practices could be taken, and which approaches are the most promising in order to make discoveries in microbiome studies more robust and reliable in the near future.
In general, I thought the titles of the big sections could be improved to better reflect their content. A few sections might require a bit of rewriting for clarification, and I would like to raise some points that are listed below.
1) The section “marker-gene sequencing”, where the case of the 16S rRNA amplicon sequencing is discussed at length (which is interesting!), is mostly dedicated to the particular task of characterizing the diversity within a community. However, it is only on lines 254-256 that the goal for using what is described as “robust marker genes” is introduced: “to characterize and compare the relative abundances of prokaryotes across communities.”
I think the 1st page of the manuscript could be re-arranged, and clarified to explain the particular usage of marker-gene approaches that is exemplified here.
- At the beginning of the section there is a discussion on the definition of a “robust marker gene”. But I believe this line of discussion depends on the goal of marker-gene sequencing – that should thus be introduced beforehand. Marker-gene approach can also be taken to question the presence of given metabolic processes in a particular environment. In which case, it is more important to fish for genes that are specifically involved in that process, leading even sometimes to multiply the set of probes to use in order to capture the diversity of the gene involved in the process of interest (some are paraphyletic for instance). In that case, the fact that the gene in question is a good molecular chronometer does not matter much, right? Or did I miss the point here?
- Line 156: a more general term would be “homolog”, as “ortholog” limits to vertically transmitted marker genes (excluding duplicated or laterally transferred genes for instance). Unless if it is explained beforehand that a desirable property of a marker gene could be to be vertically inherited? Or is the term “ortholog” used here to suggest a conserved biological function? Please clarify.
- In the end, I have the feeling that the first part of this 1st section kind of falls flat, as the authors write on lines 200-201: “Therefore, to select a robust marker gene one should adhere in some ways to the Goldilocks principle: some nucleotide conservation is needed, but not too much.” Maybe could this first part be shortened and be more straight-forward?
2) Lines 270-272: Please clarify what you mean by “V4-V5 region overrepresented Firmicutes … while drastically underestimating Actinobacteria”. Do you mean that these regions are not present from Actinobacteria? Or that the diversity is over-estimated in Firmicutes and under-estimated in Actinobacteria based on this region? Same comment for line 290-291 for V1-V2 region.
3) In the section “Shotgun Metagenomics Sequencing”, I felt like the topic of the contribution of MGS approach and MAG (metagenome assembled genomes) reconstruction to explore extant biodiversity was somehow missing (CPR, DPANN, Asgard archaea…). MGS helped to reveal novelties both at the taxonomic and functional level. As a conceptual advantage of the MGS approach, in spite of some biases highlighted by the authors, is that it is not needed to have an a priori of what is looked for. This is how some entire clades of archaea were missed by 16S approaches because of the probes being designed from known diversity (e.g. Raymann et al 2017, mSphere).
- On lines 443-447 an example is given for taxa represented in 16S data but not MGS. To be fair, the converse is also true. I don’t say the authors do not explicitly mention that there are caveats with both approaches, but this is one could be worth to be reminded.
- On lines 1004-1013, it could be added that techniques to bin MGS data as MAG could be a part of the solution.
4) On “the concordance of differential abundance results between actual and predicted metagenomics profiles” (lines 1882-1294), any lead on why the results are agreeing only “moderately well”?
5) Just a suggestion… Some figures could have been added to illustrate some parts of the text.
- On lines 1222-1225, the principle on which relies PICRUSt for inferring function is introduced. It could have been illustrated by a figure.
- On lines 1409-1412, “stacked barplots” are mentioned to be used to study functional shifts. Such a typical plot could have been borrowed from a published study for instance?
6) In the Discussion part, it would have been interesting to have the authors opinions on the role that could play new sequencing techniques in the future to help with some of the issues presented? For instance, on the advent of long-reads sequencing for MGS? Don’t you think it could eventually be a way to integrate taxonomic and functional analyses, by linking for instance 16S genes to big contigs, obtaining better quality MAGs, etc…?
7) Minor points and typos:
- A list of abbreviations should be included to help the reader. Otherwise, some of the less used abbreviations could be abandoned?
- Line 158: should it be “twice” instead of “double”?
- Line 1441 (and thereafter): maybe capitalize the tool name “phylogenize” to make it stand as a name in the text?
- Line 1445: “a taxa” => should be corrected by “a taxon”.
https://doi.org/10.24072/pci.genomics.100049.rev11Reviewed by Rafael Cuadrat, 12 Mar 2021
This review addresses many of the technical issues in the microbiome field. The text is very clear and concise, and it is very interesting for both initiated and uninitiated readers.
In general, the main point of the MS is the challenge of integrating taxonomic data with functional data. I agree that this is an issue but I feel in general the review downplay too much the binning/MAG approach dealing with this issue. I also missed in the text any discussion regarding long reads and how the 3rd generation sequencing methods could help with some of the limitations.
I have a few small comments that could improve the final version of the MS.
Line 107: There is often more statistical power to detect overall differences based on alpha and beta 108diversity metrics than to detect associations with individual features, but diversity-level insights are also less actionable (Shade 2017).
- However, often the difference of abundance in individual taxa/rank is larger than the difference in diversity indexes, especially in host-microbiome studies.
Line 422: This interest has culminated in the generation of enormous MGS datasets such as the ongoing work on the Earth Microbiome Project (Thompson et al. 2017) and the Human Microbiome Project (Lloyd-Price et al. 2017).
- Here another good and more recent example would be TARA oceans.
Line 548: “genes are expressed in cells, not in a homogenized cytoplasmic soup" (McMahon 2015).
- Agreed, however many ecological functions are performed in a collaborative way by consortiums.
Line 670: relative abundances by the mean relative abundance
- Should read geometric mean.
Line 723: This discussion of microbiome data characteristics has focused on taxonomic features based on either 16S sequencing or read-based MGS data analysis. However, it is important to emphasize that count tables produced from MAGs do not resolve this issue. In fact, attempting to account for these challenging characteristics of microbiome count data and the links between taxa and function makes the analysis more difficult.
- At the end of this, I would suggest a few lines about the network of co-abundances, for example using the SparCC tool.
https://doi.org/10.24072/pci.genomics.100049.rev12
Reviewed by Nicolas Pollet, 20 Mar 2021
Dear Gavin Douglas and Morgan Langille,
In this review manuscript, you propose to deliver a detailed introduction to microbiome DNA sequence data types and analysis methods. You present marker-gene and shotgun DNA sequencing data types, discuss microbiome data characteristics and underscore the associated caveats. Then you present « the many-faceted concept of microbial functions ». You follow by a discussion on the problematic of functional annotation inferred from marker-gene data and you review the last development on the integration of taxonomy and function. Finally, you discuss reproducibility in microbiome research and provide an outlook with some personal experience.
The main strength of your manuscript is that as a reader I learned something because you deliver an interesting review and discussion on the integration of taxonomic and functional microbiome data, backed up by first-hand and authoritative experience. However, the main weakness is that your message is diluted by a lengthy and unclear explanation of some concepts that are not always directly linked to your main discussion point.
Therefore, I recommend a major revision of your manuscript.
Sincerely,
Nicolas Pollet
Major comments:
What is the audience ? The title says « A primer and discussion on DNA-based microbiome data and related bioinformatics analyses ». Since one aim is to deliver a primer, the reader is expected to be a non-expert, and therefore the discussion that follows is also expected to reach a non-expert in the field. Is this the case ? I don’t think so. In fact, I am unsure of the efficiency to pursue the goal of fulfilling the role of a primer AND a discussion on microbiome data for a reader completely new to the field and for the complicated topics presented here. Since the reader could be misled by the title, I think you need to change it to better represent the content of the text.
Is the communication clear enough for a newcomer ? I think that you have to work on making your text more concise and more homogenous in terms of the depth of explanations. More and better iconography would help in this regard. The iconography should follow the main organization of the text : here you have six sections and only two figures. Figure 1 illustrates many aspects of the section on shotgun metagenomics, and figure 2 is an illustration on the integration of taxonomy and function. Figure 1d does not follow the text flow and I find this a bit strange.
What is the review message ? In my opinion, the discussion on the integration of taxonomic and functional data is the main message. I advise you to strengthen this aspect by dropping some sections (see below).
How to make the message clearer ? If you decide to follow the path of considering the integration of taxonomic and functional data as the main message to deliver, then the text could be reorganized to make this message stronger and clearer. I wonder if the sometime high level of details provided regarding marker-gene sequencing, shotgun metagenomics and the characteristics of microbiome count data is really helping the reader. The text would benefit from being way more concise an more equilibrated among sections. In my opinion, you should seriously consider to skip the “primer” sections on marker-gene sequencing, metagenomic sequencing, characteristics of microbiome count data and microbial functions.
I found that the discussion is the best part of the text, maybe because I am not a complete newcomer to the field. Your personal account is worthy, and maybe you could make it more precise (e.g. parameter choice from local to global using which tool ?). The last two sections are the most informative parts and in this regard.
Accuracy : The terminology about microbiome is sound and corresponds to what has been previously discussed in the literature (Marchesi & Ravel, 2015). I found that the terminology used in the section microbial function is not always clear and does not simplify the presentation of the associated concepts (Karp, 2000)(Thomas, Mi & Lewis, 2007)(Kotera et al., 2014).
Level of referencing : There are specific experimental approaches such as epicPCR that have been developed to tackle the integration of taxonomy and function; and this needs to be pointed out (Spencer et al., 2016). I think you should take a particular attention to be more homogeneous in the way you select the cited references.
Minor comments
Since the review aims to deliver a detailed introduction, I suggest to expand a bit the terminology and definition that you provide rapidly for the term microbiome (one sentence on line 31-33), and possibly include a text-box with definitions. Maybe the ecological suffix -biome that refers to biotic and abiotic factors characterizing a given microbiome environment would broaden the scope.
I fully understand that the topic is DNA-based sequencing for microbiome studies, but a pointer to RNA-based and protein-based sequencing would be a plus in the background, especially in the paragraph 45-67. In that same paragraph on culturing microbes, and given the theme of the integration between taxonomy and function, one possible additional point could be to discuss the discrimination of live, dormant and dead microorganisms (e.g. (Thomas, Mi & Lewis, 2007) (Jones & Lennon, 2010)(Carini et al., 2016)(Blazewicz et al., 2013).
In the background section presenting diversity analysis, I would like to underscore the work of Amy Willis and colleagues on modelling abundances as in my opinion it is an important advance in the analysis of diversity (Willis, 2019)(Willis & Martin). The purpose of this paragraph in the context of the review as a whole is unclear as it stands.
I do not agree with the assertion that the dichotomy between phylogenetic and functional profiling of microbiomes is « entirely related to methodological challenges » (line 123). We know that the genome of prokaryotic species varies in gene content because of horizontal gene transfer, gene duplication and other mechanisms (Puigbò et al., 2014). It has been shown through pangenome analysis that strain variation can be associated with different metabolic potential (Goyal, 2018) (Maistrenko et al., 2020). Therefore, it seems to me that the dichotomy between phylogenetic and functional profiling of microbiomes is one of their intrinsic characteristics. Indeed, you develop these points line 1131-1172.
Marker-gene sequencing
I advise to simplify the marker gene sequencing section if you want to keep it. While the paragraph from 149-202 are detailed and very informative, I am afraid that they depart from the global « granularity » of explanation and historical context provided on other aspects throughout the manuscript. This lengthen this section on marker genes comparatively to the other aspects developed in this review. And even if there are a lot of things to tell about 16S rRNA gene sequencing, many have already been told elsewhere in the literature.
While I typically enjoy reading historical perspectives, I found that these are exaggeratedly long and placed in the manuscript in a non-logical manner.
You copiously present 16S rRNA gene sequencing and this helps the reader for understanding the aspects on the integration of taxonomic and functional data. But you also consider other marker genes (and this is fine) and 18s rRNA gene sequencing for microeukaryote and fungi taxonomic profiling, but in a more concise manner. Yet the integration of taxonomic data obtained using such markers with shotgun sequencing data is not presented at all, and thus the reader does not benefit from this otherwise interesting piece of knowledge.
The sentence line 211 would benefit from some simplification such as :
« This is because if there are non-random substitutions within a single domain but random substitutions in the majority of other domains, there would likely be little effect on estimates of gene divergence. »
I do not understand the reason for presenting redbiom at this point line 250 ?
To further document your point on the limitations due to the use of short 16S amplicons (line 260-274), you could possibly cite the recent work of other groups such as (Abellan-Schneyder et al., 2021).
The point dealing with the use of classical bacteria 16S primer-pairs do characterize Archaea could be expanded as it is often a neglected limitation in taxonomic surveys (Raymann et al., 2017; Bahram et al., 2019).
The reference Fox et al 1992 is missing at line 235. I think it would be fair to reference deblur and UNOISE3 like it has been made for DADA2 software (line 336).
Very Minor : italicize latin names (e.g Haloarcula line 382)
Shotgun metagenomics sequencing
Line 409 : including DNA viruses
The impact of biomass and genome size as a limitation to MGS approach could be invoked (line 431). Also as a caveat emptor, the impact of host DNA and possible heterologous sequences on MGS data could be mentioned, (I wrote this sentence before reading your discussion !) and this would be a reflection of the discussion.
In the MGS data analysis section devoted to the generation of taxonomic profile (line 477-522) , I would like to point out the targeted assembly of rRNA sequences from shotgun data embodied in Emirge (Miller et al., 2011), phyloFlash (Gruber-Vodicka, Seah & Pruesse, 2020) and MATAM (Pericard et al., 2018).
I was surprised that the authors do not mention Kaiju as a read-based tool for taxonomic profiling (Menzel, Ng & Krogh, 2016).
On the impact of databases for k-mer based analysis (Nasko et al., 2018).
Line 560 : the citation of only these two assemblers is somehow partial, you could point to a review on metagenome assembly for the sake of comprehensiveness for the reader. Similarly the description of binning tools is very light in comparison to other aspects developed earlier. Here you could point to recent review papers on the subject.
Line 584 : maybe use « taxonomic profiling » instead of « profiling »
Line 586 : I guess that the authors are referring to transcriptome studies, the term RNA sequencing is maybe not so precise in this context.
Characteristics of microbiome count data :
Maybe at some point the word abundance table could be used.
Line 618-637 : Maybe a figure would be a better communication vector.
The impact of sequencing reads processing on the analysis of abundance tables is somehow skipped : there are different practices such as removing singletons, filtering on prevalence etc . This could be somehow mentioned as they impact downstream analysis.
Microbial functions
This section is quite lengthy in comparison to others and since it covers topics that are not specific to microbiome studies, I wonder if it hits the sweet spot.
Line 737 : « … focused on gene families, which are gene clusters. » It is not very clear what you are referring to in terms of gene cluster at this point.
Line 781 : I do not know what is a UniRef function.
What is described in this paragraph entitled microbial function is in fact a primer on protein databases and ontologies. I find therefore that the title is a bit misleading, maybe « Protein databases and ontologies for microbial genome functional annotation ».
Line 976 : this method focuses pathway reconstruction … please correct the sentence.
Line 1032 : philosophical perspective : really ?
Line 1060 : The whole presentation of this paragraph is somehow paradoxal : maybe the text could be more explicit on ontology and semantics in order to guide the analysis of « functional data » at a given level of an ontology (protein space, biochemical activity, pathway, evolutionary conservation.
Metagenome prediction methods
Line 1090-1097 : some references would be welcome here.
Lines 1101-1110 -1130: This historical account is perfect, but I wonder if the level of details provided is really needed to make the point that 16S diversity is not a perfect proxy of whole genome similarity.
Current state of the integration of taxonomic and functional data types
I enjoyed reading this section.
Line 1313: “in some cases can be directly linked” Please be more precise and provide an example or a reference.
Why the burrito software is not mentioned is unclear to me ?
Outlook
In my opinion, the paragraph 1726-1761 would benefit from citing additional recent references such as the MBQC study and a few others: (Sinha et al., 2017; Davis et al., 2018; McLaren, Willis & Callahan, 2019; Greathouse, Sinha & Vogtmann, 2019).
References
I suggest to use a style for references that includes a DOI.
Abellan-Schneyder I, Matchado MS, Reitmeier S, Sommer A, Sewald Z, Baumbach J, List M, Neuhaus K. 2021. Primer, Pipelines, Parameters: Issues in 16S rRNA Gene Sequencing. mSphere 6. DOI: 10.1128/mSphere.01202-20.
Bahram M, Anslan S, Hildebrand F, Bork P, Tedersoo L. 2019. Newly designed 16S rRNA metabarcoding primers amplify diverse and novel archaeal taxa from the environment. Environmental Microbiology Reports 11:487–494. DOI: 10.1111/1758-2229.12684.
Blazewicz SJ, Barnard RL, Daly RA, Firestone MK. 2013. Evaluating rRNA as an indicator of microbial activity in environmental communities: limitations and uses. The ISME journal 7:2061–2068. DOI: 10.1038/ismej.2013.102.
Carini P, Marsden PJ, Leff JW, Morgan EE, Strickland MS, Fierer N. 2016. Relic DNA is abundant in soil and obscures estimates of soil microbial diversity. Nature Microbiology 2:1–6. DOI: 10.1038/nmicrobiol.2016.242.
Davis NM, Proctor DM, Holmes SP, Relman DA, Callahan BJ. 2018. Simple statistical identification and removal of contaminant sequences in marker-gene and metagenomics data. Microbiome 6:226. DOI: 10.1186/s40168-018-0605-2.
Emerson JB, Adams RI, Román CMB, Brooks B, Coil DA, Dahlhausen K, Ganz HH, Hartmann EM, Hsu T, Justice NB, Paulino-Lima IG, Luongo JC, Lymperopoulou DS, Gomez-Silvan C, Rothschild-Mancinelli B, Balk M, Huttenhower C, Nocker A, Vaishampayan P, Rothschild LJ. 2017. Schrödinger’s microbes: Tools for distinguishing the living from the dead in microbial ecosystems. Microbiome 5:86. DOI: 10.1186/s40168-017-0285-3.
Goyal A. 2018. Metabolic adaptations underlying genome flexibility in prokaryotes. PLOS Genetics 14:e1007763. DOI: 10.1371/journal.pgen.1007763.
Greathouse KL, Sinha R, Vogtmann E. 2019. DNA extraction for human microbiome studies: the issue of standardization. Genome Biology 20:212. DOI: 10.1186/s13059-019-1843-8.
Gruber-Vodicka HR, Seah BKB, Pruesse E. 2020. phyloFlash: Rapid Small-Subunit rRNA Profiling and Targeted Assembly from Metagenomes. mSystems 5. DOI: 10.1128/mSystems.00920-20.
Jones SE, Lennon JT. 2010. Dormancy contributes to the maintenance of microbial diversity. Proceedings of the National Academy of Sciences 107:5881–5886. DOI: 10.1073/pnas.0912765107.
Karp PD. 2000. An ontology for biological function based on molecular interactions. Bioinformatics (Oxford, England) 16:269–285. DOI: 10.1093/bioinformatics/16.3.269.
Kotera M, Nishimura Y, Nakagawa Z, Muto A, Moriya Y, Okamoto S, Kawashima S, Katayama T, Tokimatsu T, Kanehisa M, Goto S. 2014. PIERO ontology for analysis of biochemical transformations: effective implementation of reaction information in the IUBMB enzyme list. Journal of Bioinformatics and Computational Biology 12:1442001. DOI: 10.1142/S0219720014420013.
Maistrenko OM, Mende DR, Luetge M, Hildebrand F, Schmidt TSB, Li SS, Rodrigues JFM, von Mering C, Pedro Coelho L, Huerta-Cepas J, Sunagawa S, Bork P. 2020. Disentangling the impact of environmental and phylogenetic constraints on prokaryotic within-species diversity. The ISME Journal 14:1247–1259. DOI: 10.1038/s41396-020-0600-z.
Marchesi JR, Ravel J. 2015. The vocabulary of microbiome research: a proposal. Microbiome 3:31. DOI: 10.1186/s40168-015-0094-5.
McLaren MR, Willis AD, Callahan BJ. 2019. Consistent and correctable bias in metagenomic sequencing experiments. eLife 8. DOI: 10.7554/eLife.46923.
Menzel P, Ng KL, Krogh A. 2016. Fast and sensitive taxonomic classification for metagenomics with Kaiju. Nature Communications 7:11257. DOI: 10.1038/ncomms11257.
Miller CS, Baker BJ, Thomas BC, Singer SW, Banfield JF. 2011. EMIRGE: reconstruction of full-length ribosomal genes from microbial community short read sequencing data. Genome Biology 12:1–14. DOI: 10.1186/gb-2011-12-5-r44.
Nasko DJ, Koren S, Phillippy AM, Treangen TJ. 2018. RefSeq database growth influences the accuracy of k-mer-based lowest common ancestor species identification. Genome Biology 19. DOI: 10.1186/s13059-018-1554-6.
Pericard P, Dufresne Y, Couderc L, Blanquart S, Touzet H. 2018. MATAM: reconstruction of phylogenetic marker genes from short sequencing reads in metagenomes. Bioinformatics 34:585–591. DOI: 10.1093/bioinformatics/btx644.
Puigbò P, Lobkovsky AE, Kristensen DM, Wolf YI, Koonin EV. 2014. Genomes in turmoil: quantification of genome dynamics in prokaryote supergenomes. BMC Biology 12. DOI: 10.1186/s12915-014-0066-4.
Raymann K, Moeller AH, Goodman AL, Ochman H. 2017. Unexplored Archaeal Diversity in the Great Ape Gut Microbiome. mSphere 2. DOI: 10.1128/mSphere.00026-17.
Sinha R, Abu-Ali G, Vogtmann E, Fodor AA, Ren B, Amir A, Schwager E, Crabtree J, Ma S, Abnet CC, Knight R, White O, Huttenhower C. 2017. Assessment of variation in microbial community amplicon sequencing by the Microbiome Quality Control (MBQC) project consortium. Nature Biotechnology 35:1077–1086. DOI: 10.1038/nbt.3981.
Spencer SJ, Tamminen MV, Preheim SP, Guo MT, Briggs AW, Brito IL, A Weitz D, Pitkänen LK, Vigneault F, Juhani Virta MP, Alm EJ. 2016. Massively parallel sequencing of single cells by epicPCR links functional genes with phylogenetic markers. The ISME journal 10:427–436. DOI: 10.1038/ismej.2015.124.
Thomas PD, Mi H, Lewis S. 2007. Ontology annotation: mapping genomic regions to biological function. Current Opinion in Chemical Biology 11:4–11. DOI: 10.1016/j.cbpa.2006.11.039.
Willis AD. 2019. Rigorous Statistical Methods for Rigorous Microbiome Science. mSystems 4. DOI: 10.1128/mSystems.00117-19.
Willis AD, Martin BD. Estimating diversity in networked ecological communities. Biostatistics. DOI: 10.1093/biostatistics/kxaa015.
https://doi.org/10.24072/pci.genomics.100049.rev13