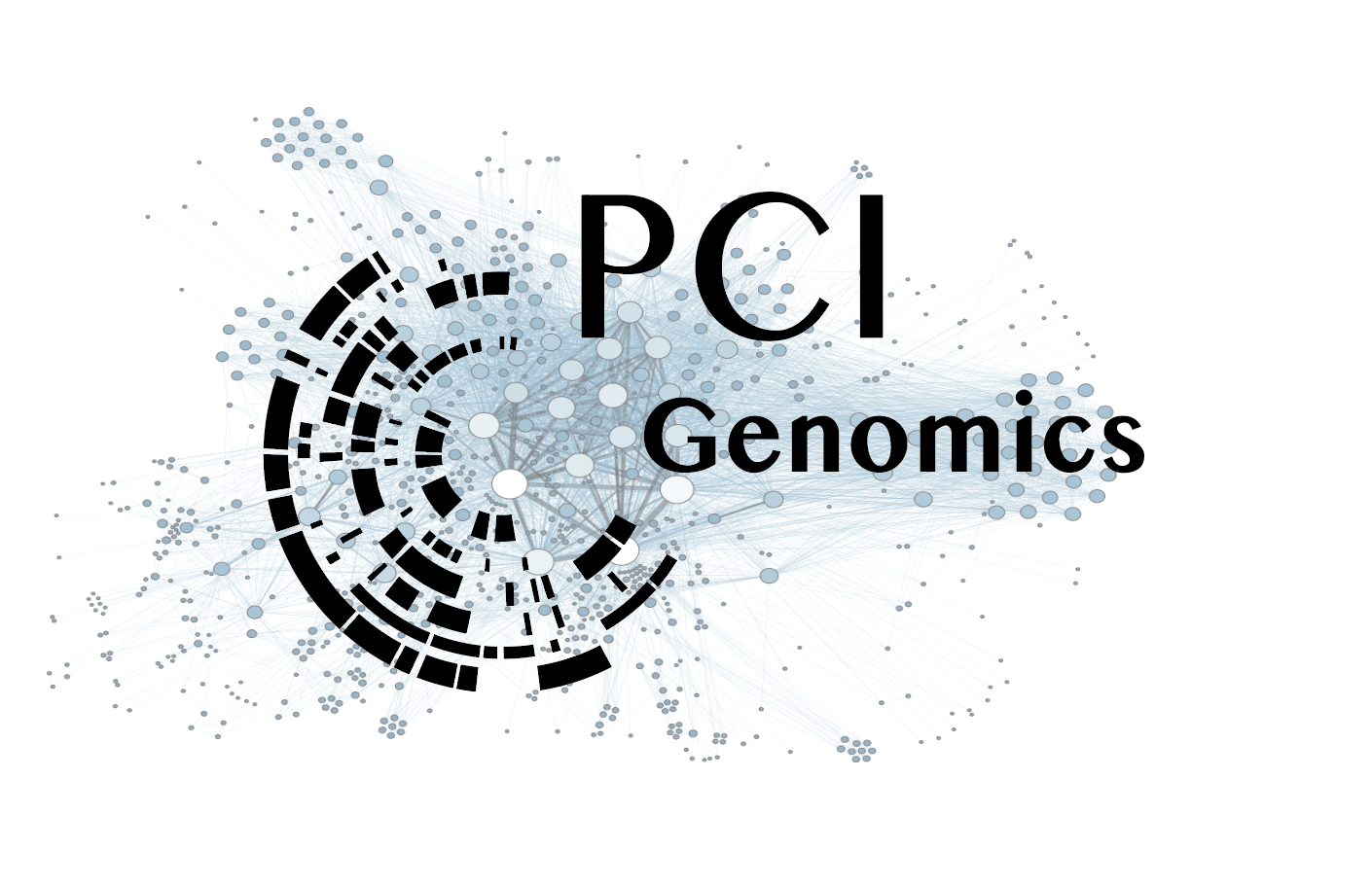
Toward a critical assessment of virus detection in plants
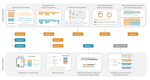
Semi-artificial datasets as a resource for validation of bioinformatics pipelines for plant virus detection
Abstract
Recommendation: posted 02 April 2021, validated 02 April 2021
Quesneville, H. (2021) Toward a critical assessment of virus detection in plants. Peer Community in Genomics, 100007. 10.24072/pci.genomics.100007
Recommendation
The advent of High Throughput Sequencing (HTS) since the last decade has revealed previously unsuspected diversity of viruses as well as their (sometimes) unexpected presence in some healthy individuals. These results demonstrate that genomics offers a powerful tool for studying viruses at the individual level, allowing an in-depth inventory of those that are infecting an organism. Such approaches make it possible to study viromes with an unprecedented level of detail, both qualitative and quantitative, which opens new venues for analyses of viruses of humans, animals and plants. Consequently, the diagnostic field is using more and more HTS, fueling the need for efficient and reliable bioinformatics tools.
Many such tools have already been developed, but in plant disease diagnostics, validation of the bioinformatics pipelines used for the detection of viruses in HTS datasets is still in its infancy. There is an urgent need for benchmarking the different tools and algorithms using well-designed reference datasets generated for this purpose. This is a crucial step to move forward and to improve existing solutions toward well-standardized bioinformatics protocols. This context has led to the creation of the Plant Health Bioinformatics Network (PHBN), a Euphresco network project aiming to build a bioinformatics community working on plant health. One of their objectives is to provide researchers with open-access reference datasets allowing to compare and validate virus detection pipelines.
In this framework, Tamisier et al. [1] present real, semi-artificial, and completely artificial datasets, each aimed at addressing challenges that could affect virus detection. These datasets comprise real RNA-seq reads from virus-infected plants as well as simulated virus reads. Such a work, providing open-access datasets for benchmarking bioinformatics tools, should be encouraged as they are key to software improvement as demonstrated by the well-known success story of the protein structure prediction community: their pioneer community-wide effort, called Critical Assessment of protein Structure Prediction (CASP)[2], has been providing research groups since 1994 with an invaluable way to objectively test their structure prediction methods, thereby delivering an independent assessment of state-of-art protein-structure modelling tools. Following this success, many other bioinformatic community developed similar “competitions”, such as RNA-puzzles [3] to predict RNA structures, Critical Assessment of Function Annotation [4] to predict gene functions, Critical Assessment of Prediction of Interactions [5] to predict protein-protein interactions, Assemblathon [6] for genome assembly, etc. These are just a few examples from a long list of successful initiatives. Such efforts enable rigorous assessments of tools, stimulate the developers’ creativity, but also provide user communities with a state-of-art evaluation of available tools.
Inspired by these success stories, the authors propose a “VIROMOCK challenge” [7], asking researchers in the field to test their tools and to provide feedback on each dataset through a repository. This initiative, if well followed, will undoubtedly improve the field of virus detection in plants, but also probably in many other organisms. This will be a major contribution to the field of viruses, leading to better diagnostics and, consequently, a better understanding of viral diseases, thus participating in promoting human, animal and plant health.
References
[1] Tamisier, L., Haegeman, A., Foucart, Y., Fouillien, N., Al Rwahnih, M., Buzkan, N., Candresse, T., Chiumenti, M., De Jonghe, K., Lefebvre, M., Margaria, P., Reynard, J.-S., Stevens, K., Kutnjak, D. and Massart, S. (2021) Semi-artificial datasets as a resource for validation of bioinformatics pipelines for plant virus detection. Zenodo, 4273791, version 4 peer-reviewed and recommended by Peer community in Genomics. doi: https://doi.org/10.5281/zenodo.4273791
[2] Critical Assessment of protein Structure Prediction” (CASP) - https://en.wikipedia.org/wiki/CASP
[3] RNA-puzzles - https://www.rnapuzzles.org
[4] Critical Assessment of Function Annotation (CAFA) - https://en.wikipedia.org/wiki/Critical_Assessment_of_Function_Annotation
[5] Critical Assessment of Prediction of Interactions (CAPI) - https://en.wikipedia.org/wiki/Critical_Assessment_of_Prediction_of_Interactions
[6] Assemblathon - https://assemblathon.org
[7] VIROMOCK challenge - https://gitlab.com/ilvo/VIROMOCKchallenge
The recommender in charge of the evaluation of the article and the reviewers declared that they have no conflict of interest (as defined in the code of conduct of PCI) with the authors or with the content of the article. The authors declared that they comply with the PCI rule of having no financial conflicts of interest in relation to the content of the article.
Evaluation round #2
DOI or URL of the preprint: https://zenodo.org/record/4273792#.YEIjC-fjKUk, https://zenodo.org/record/4584967#.YEIku-fjKUk
Version of the preprint: 10.5281/zenodo.4273792, 10.5281/zenodo.4584967
Author's Reply, 02 Apr 2021
The two references have been added
Decision by Hadi Quesneville, posted 15 Mar 2021
The responses brought by the autors to the reviewers are satisfactory. However two references in the text "Text S1" (line 117) and "Table S1" (line 125) cannot be found in the manuscript. The authors should fix this in order to have their preprint recommended.
Evaluation round #1
DOI or URL of the preprint: https://zenodo.org/record/4293594#.X8D6GLPjJEY
Author's Reply, 05 Mar 2021
Decision by Hadi Quesneville, posted 19 Jan 2021
Dear authors,
The two referees found your article interesting and potentially of great value. However, it can be still improved according to their suggestions. I recommend you to take into account their suggestions and to re-submit it for a second evaluation round.
Best regards,
Hadi Quesneville
Reviewed by anonymous reviewer 1, 18 Dec 2020
In this manuscript, the authors aim at describing several semi-artificial and artificial dataset of plant virus that could be used to benchmark bioinformatic pipelines for virus identification, allowing the assessment of their performance.
The initiative is very commendable and truly necessary with the number of bioinformatics tools developed today in all fields of biology. However, I have a real problem with this manuscript which seems to me insufficiently accomplished with a lack of information and precision.
The subject of the article is very specialized as it concerns the detection of plant viruses, this is why it is important to better introduce the subject.
There is a problem in the lack of explanation concerning the type of data allowing these detection or how they are obtained (from which biological data). Are they RNA-seq or DNA-seq data, or both? Do they come from purified extract from tissues (meaning are there steps of filtration to enrich in virus sequences or is there also host sequences)?
Likewise, it would be desirable to recall the existing bioinformatic tools or at least the approaches used depending on the questions asked to have an idea about the difficulties of these approaches.
The proposed dataset are also not very detailed nor the way they have been constructed. Especially concerning the real data. Sometimes figures would be useful to illustrate the text.
Another missing point is the lack of proof of principle to show examples in the use of at least some of these dataset and how they really allow a good benchmarking process.
Finally, the authors argue about the fact that having semi-artificial dataset allow to bypass the drawbacks of having either only real dataset or completely artificial dataset. This seems contradictory with the fact that the authors propose 3 real dataset and 9 artificial ones among the 18 dataset. Moreover, I think the semi-artificial dataset may also have some drawbacks that could be discussed. It could be possible that the drawbacks of both artificial and real dataset add up.
In sum, I think this work is needed since benchmarking bioinformatic tools is of utmost importance. However, this manuscript does not meet, at this stage, standards of scientific publications.
Reviewed by Alexander Suh, 19 Jan 2021
Tamisier et al. provide a combination of real and semi-artificial datasets with high relevance for benchmarking detection and analysis approaches in plant virus detection. The manuscript is succinct and well written, accompanied by a detailed GitLab repository, and proposes the VIROMOCK challenge as a community-driven effort to benchmark virus detection and analysis.
Below are some minor suggestions for improved clarity that the authors may want to implement to help a broad readership.
- Line 86: It is unclear whether the read lengths vary within or between each data set. Table 1 suggests that the latter is mostly the case, however, then it would help the reader if the distinct sets of read lengths were stated here in the text.
- Lines 91-94: Both for the real and artificial dataset, I recommend briefly discussing the potential issues arising from Illumina's recent shift from a four-channel system (e.g., HiSeq X) to a two-channel system (e.g. NovaSeq). A recent opinion piece by De-Kayne et al. (https://onlinelibrary.wiley.com/doi/epdf/10.1111/1755-0998.13309) reviewed evidence for T>G errors in NovaSeq data and provided suggestions for how to deal with this. I assume this does not affect the datasets presented in the present manuscript (assuming all data here are based on HiSeq data or simulated on these), but this may be important to be pointed out for readers using NovaSeq data or HiSeq/NovaSeq combinations after benchmarking with the present datasets. Please also clarify in the text what system the present datasets are based or simulated on.
- Line 101: Here and throughout, it may be unclear to some readers whether "non-complete genome" refers to the virus or the host.
- Line 113: I commend the authors on preparing a very detailed GitLab repository. The Dryad download links appear to be working here, unlike the DOIs stated in Table 1. Please make sure that the DOIs stated in Table 1 are accessible, I was unable to have a look at the datasets through the Table 1 DOI links.
- Line 145: Did the authors double-check that the random removal of reads led to complete absence of coverage for some genomic regions of these viruses, rather than reduced coverage for these regions?
- Line 216: I like the diversity of challenging datasets discussed in the text and the authors' idea for the VIROMOCK challenge, however, for visual learners it might help to summarize key points in a figure. If the authors agree that this would help, consider providing simplified illustrations of virus detection/analysis challenges (with pointers to datasets 1-18), and/or the suggested community-driven approach of the VIROMOCK challenge.
- Table 1: In the modification column, consider stating the number of reads (or read pairs) added, and possibly also the number of strains.
- Table 1: In the "Challenge" column, it is not always clear which virus a specific "mutation" or "strain" refers to. Please revise for clarity by adding as much information as space allows.