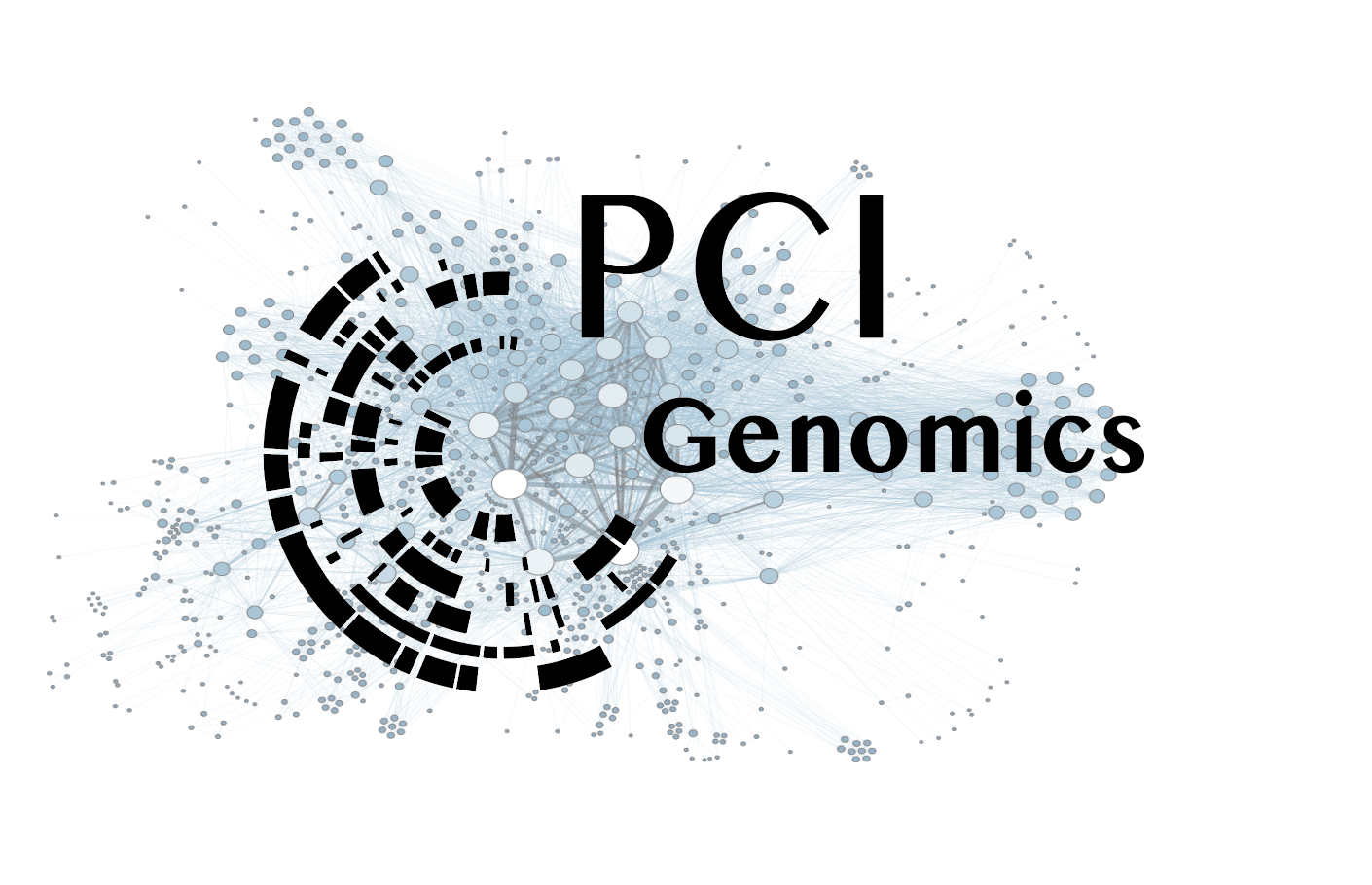
A pipeline to select SARS-CoV-2 sequences for reliable phylodynamic analyses
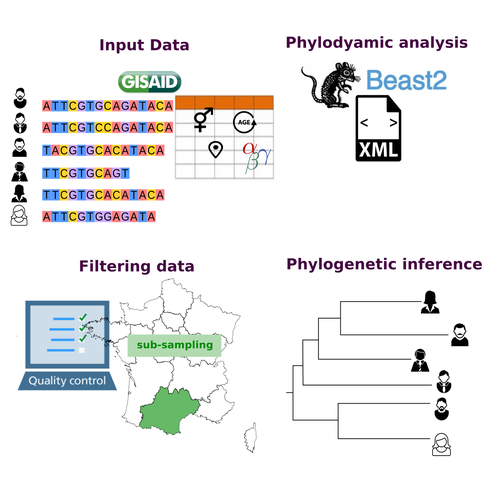
COVFlow: phylodynamics analyses of viruses from selected SARS-CoV-2 genome sequences
Abstract
Recommendation: posted 07 September 2023, validated 11 September 2023
Lerat, E. (2023) A pipeline to select SARS-CoV-2 sequences for reliable phylodynamic analyses. Peer Community in Genomics, 100239. 10.24072/pci.genomics.100239
Recommendation
Phylodynamic approaches enable viral genetic variation to be tracked over time, providing insight into pathogen phylogenetic relationships and epidemiological dynamics. These are important methods for monitoring viral spread, and identifying important parameters such as transmission rate, geographic origin and duration of infection [1]. This knowledge makes it possible to adjust public health measures in real-time and was important in the case of the COVID-19 pandemic [2]. However, these approaches can be complicated to use when combining a very large number of sequences. This was particularly true during the COVID-19 pandemic, when sequencing data representing millions of entire viral genomes was generated, with associated metadata enabling their precise identification.
Danesh et al. [3] present a bioinformatics pipeline, CovFlow, for selecting relevant sequences according to user-defined criteria to produce files that can be used directly for phylodynamic analyses. The selection of sequences first involves a quality filter on the size of the sequences and the absence of unresolved bases before being able to make choices based on the associated metadata. Once the sequences are selected, they are aligned and a time-scaled phylogenetic tree is inferred. An output file in a format directly usable by BEAST 2 [4] is finally generated.
To illustrate the use of the pipeline, Danesh et al. [3] present an analysis of the Delta variant in two regions of France. They observed a delay in the start of the epidemic depending on the region. In addition, they identified genetic variation linked to the start of the school year and the extension of vaccination, as well as the arrival of a new variant. This tool will be of major interest to researchers analysing SARS-CoV-2 sequencing data, and a number of future developments are planned by the authors.
References
[1] Baele G, Dellicour S, Suchard MA, Lemey P, Vrancken B. 2018. Recent advances in computational phylodynamics. Curr Opin Virol. 31:24-32. https://doi.org/10.1016/j.coviro.2018.08.009
[2] Attwood SW, Hill SC, Aanensen DM, Connor TR, Pybus OG. 2022. Phylogenetic and phylodynamic approaches to understanding and combating the early SARS-CoV-2 pandemic. Nat Rev Genet. 23:547-562. https://doi.org/10.1038/s41576-022-00483-8
[3] Danesh G, Boennec C, Verdurme L, Roussel M, Trombert-Paolantoni S, Visseaux B, Haim-Boukobza S, Alizon S. 2023. COVFlow: phylodynamics analyses of viruses from selected SARS-CoV-2 genome sequences. bioRxiv, ver. 7 peer-reviewed and recommended by Peer Community in Genomics. https://doi.org/10.1101/2022.06.17.496544
[4] Bouckaert R, Heled J, Kühnert D, Vaughan T, Wu C-H et al. 2014. BEAST 2: a software platform for Bayesian evolutionary analysis. PLoS Comput Biol 10: e1003537. https://doi.org/10.1371/journal.pcbi.1003537
The recommender in charge of the evaluation of the article and the reviewers declared that they have no conflict of interest (as defined in the code of conduct of PCI) with the authors or with the content of the article. The authors declared that they comply with the PCI rule of having no financial conflicts of interest in relation to the content of the article.
This project was supported by the Agence Nationale de la Recherche Maladies Infectieuses Émergentes to the MODVAR project (grant no. ANRS0151)
Evaluation round #3
DOI or URL of the preprint: https://doi.org/10.1101/2022.06.17.496544
Version of the preprint: 3
Author's Reply, 06 Sep 2023
Dear Dr. Lerat,
As suggested the title has been changed to "COVFlow: phylodynamics analyses of viruses from selected SARS-CoV-2 genome sequences".
Best,
-Gonché Danesh and co-authors
Decision by Emmanuelle Lerat, posted 04 Sep 2023, validated 05 Sep 2023
Dear Dr. Danesh,
Thank you for submitting a revised version of your manuscript.
Before I can make my final recommendation, could you slightly change the title of your manuscript?
Could you use one of the following alternatives “COVFlow: viral phylodynamics analyses from selected SARS-CoV-2 genome sequences” or “COVFlow: phylodynamics analyses of viruses from selected SARS-CoV-2 genome sequences”. The second one may be better due to the increase in the non-biological reference to the term “viral” in recent years, however both are suitable.
Sincerely,
Emmanuelle Lerat
Evaluation round #2
DOI or URL of the preprint: https://doi.org/10.1101/2022.06.17.496544
Version of the preprint: 2
Author's Reply, 20 Jul 2023
Decision by Emmanuelle Lerat, posted 13 Jul 2023, validated 13 Jul 2023
Dear Dr Gonché,
Thank you for the revised version of your manuscript. Only few minor points remained to be done before I can recommend your article. Please consider carefullly the propositions of the reviewer.
Sincerely
Emmanuelle Lerat
Reviewed by Gabriel Wallau
, 08 Jul 2023
Danesh and collaborators reviewed the manuscript adding and adjusting parameters of the COVFflow pipeline, clarifying some sections, highlighting some of the limitations of the pipeline configurations and analysis along with more robust analyses. Therefore, I recomment its acceptance after minor edits as below.
More specifically, the authors improved the results section regarding the comparison with nextstrain. I recommend the authors to include the information present in one of their answers: "For example, it can select data if a column contains a certain word, allowing the user to filter data that may contain spelling mistakes or to select data from a group of laboratories that contain a common word (in our case CERBA) but don’t have the same names". Only including "COVflow allows a more flexible filtering stage using the JSON file" (page 8 - line 169) don't make it clear.
The authors also created a test dataset and updated the workflow documentation accordingly. There is a divergence in documentation and the test data. The test files zip on repository are covflow_test_dataset.zip that englobes covflow_test_metadata.tsv and covflow_test_sequences.fasta and in the documentation is informed: "In the data directory, the compressed archive data_test.zip contains a fasta file (sequences.fasta) and tsv file (metadata.tsv)." So I recommend the authors to correct the name of test files, or the documentation.
Page 8 line 166 - change Nextrain to Nextclade
Page 30 line 261 - “from raw sequence data to phylodynamics analyses.” its looks like that covflow perform raw sequence reads analysis and genome assembly which is not the case. Please correct it.
Evaluation round #1
DOI or URL of the preprint: https://doi.org/10.1101/2022.06.17.496544
Version of the preprint: 1
Author's Reply, 12 Jun 2023
Decision by Emmanuelle Lerat, posted 06 Mar 2023, validated 08 Mar 2023
Dear Dr Gonché,
I have now received the comments of two reviewers for your manuscript. They both find your work very interesting but point some important issues that need to be addressed, especially the lack of data test. Moreover, reviewer 1 had difficulty accessing the program.
Sincerely
Emmanuelle Lerat
Reviewed by Bastien Boussau, 05 Mar 2023
Danesh et al. present a pipeline to select sequences according to a range of criteria from data downloaded from Gisaid. It can be used to select sequences from a specific range of dates, from specific regions, from specific sequencing laboratories, from specific viral lineages, by specifying the options in a yaml file. Further filtering options can be used as well according to a json file. Once the data has been filtered, the pipeline can align the sequences, construct and date a phylogeny, and build the configuration file for a BEAST2 birth-death skyline plot analysis. The manuscript describes the pipeline, and presents an example analysis that has been performed with the pipeline.
The manuscript is clear and easy to read. The tool provided is likely to be useful, is easy to install (although I report below one typo), with a very good documentation, but it is somewhat hard to test, because the authors have not provided a test data set. This is likely due to GISAID rules, which prevent distributing subsets of their data. However, the authors could build a mock data set, with a mock aligment (which can be very small), and mock metadata, on which their pipeline could run. This example might be useful to users who first want to try the tool on a small data set before they try it on their own.
Another recommendation I have would be to comment on the importance of the different priors users have to set for the parameters of the BDSKY analysis (in the minor comments below I point out that the "origin" parameter might be an important one). Some parameters may have a stronger influence than others, and their impact on the analysis may not be obvious to users. This information could be provided on their gitlab website.
Minor comments:
l22: "were made available" : have been made available
l23: "This allowed" : has allowed
l31: "go from dates virus sequence data": dated
l122: "This yields infectious periods varying from 1.2 to 36.5 days": perhaps specify the mean, and clarify a bit because the parameter was specified a couple of lines above as per year, whereas this sentence is in days, which can be a bit confusing.
l128: "The default prior for this parameter prior is a uniform distribution Uniform(0, 2) years.": this prior seems a bit dangerous for naive users who may be using the method in the future. If they don't change it, it seems like they would not be able to infer origin dates older than 2 years from the date of their analysis.
Fig. 2 legend: "In panel c, the lined show" : lines
l155: "allow us to visualise": allows us
l161: "variant epidemic seems to occurred earlier and more frequently": have occurred
l168: "PACA experience a period of Delta variant growth": experienced
l180: "from the GISAID datased" : dataset
Software test:
cd cov-flow : cd Cov-flow
Reviewed by Gabriel Wallau
, 30 Jan 2023
Danesh and collaborators presented COVFlow, a computational pipeline aimed to perform sample selection and phylodynamic analysis of SARS-CoV-2 sequences. Due to the huge amount of SARS-CoV-2 sequences available in public databases such pipelines are in much need to select datasets that are amenable to computational analysis and inferences. Therefore, COVFlow addresses an important bottleneck in the field of genomic surveillance particularly regarding the generation of virus transmission rate inferences that is a key information to inform the public health decision making process. However, from the application point of view this pipeline is able to perform similar steps already performed by other highly used software (i.e. Nextclade). In addition, I could not test the pipeline due to user permission restriction. In summary, I suggest a number of modifications and clarifications in the manuscript to be able to reassess its in more in detail.
Comments and requests
Page 3 - line 31 - I suggest changing “dates virus sequence data” to “data stamped virus sequence data.”
page 3 - lines 40-41. What authors meant with “However, these do not include a data filtration step based on metadata characteristics.”? The nextstrain CLI tool, which includes Augur in some steps, allows the user to filter data based on different metadata (see https://docs.nextstrain.org/projects/ncov/en/latest/guides/workflow-config-file.html), such as: collection date, pangolin lineage, genome length, host, geographic information (region, country, division, location). I suggest the authors clarify which metadata COVFlow can filter out that nextclade can not. Moreover, I recommend the authors to describe the advantages of each step of CovFlow (filtering, alignment, masking sites and build tree) when compared with nextstrain (https://docs.nextstrain.org/en/latest/learn/parts.html). From my point of view there are two new COVFlow features compared with nextstrain CLI, that is, subsampling appears to be a proportional sampling in the models (instead of a absolute number per sampling group model that can be set in nextclade) and the generation of XML file to be used on beast2.
Page 6 - line 96 - Why the authors used the option “and ’addfragments’” if the sequences are almost all full length? Maybe the --add option is enough. Please clarify.
Page 7-9 - lines 137-152 - Regarding the selection of samples for BDSKY analysis, one key step is the selection of monophyletic clades and then performing Re estimates on them separately. Otherwise, Re estimates could be much biased by inferring transmission timing dynamics from unstable “clades”, which means that every run of the pipeline may generate a different time-tree structure and reach different Re estimates. Did the authors include such a step on the pipeline? Please clarify.
Moreover, figure 2 lower section should be depicted with case numbers from each region and the whole country to evaluate if the Re estimates are compatible with the epidemiological curves. I suggest three different plots, one for each region considered.
At the moment of Delta variant spread the population had already a complex mix of acquired and vaccine induced immunity. It would be interesting to add the vaccination rate from each region through time in this figure as well.
Page 9 - lines 168-171. Are there any other available genomic data that could provide some additional lines of evidence of a Delta growth at this time point besides inference tests? Proportion of genomic defined lineages? One suggestion is to plot the lineage GISAID data itself from each region and France alongside Figure 2C.
Git Lab issues
Following the Gitllab instructions on installing COVflow, the git clone section returns: fatal: Could not read from remote repository.
The authors should clarify how to obtain the tsv metadata file. Can it be obtained from the general metadata present on the Download section of GISAID - EpiCov or it came from the metadata available after a sequence selection performed in the search interface of GISAID - EpiCov? If the metadata file has more columns than the ones specified on Metadata Fields would COVFlow still work?
I suggest that the authors create a test dataset with fasta and metadata files or inform a way that the user can recover it from Gisaid and an associated step-by-step guide that could be followed by the user to perform a test analysis with the current json files present in examples directory. This will facilitate the user implementation of COVFlow through simple testing.